Transforming Autonomous Driving with Semantic Segmentation
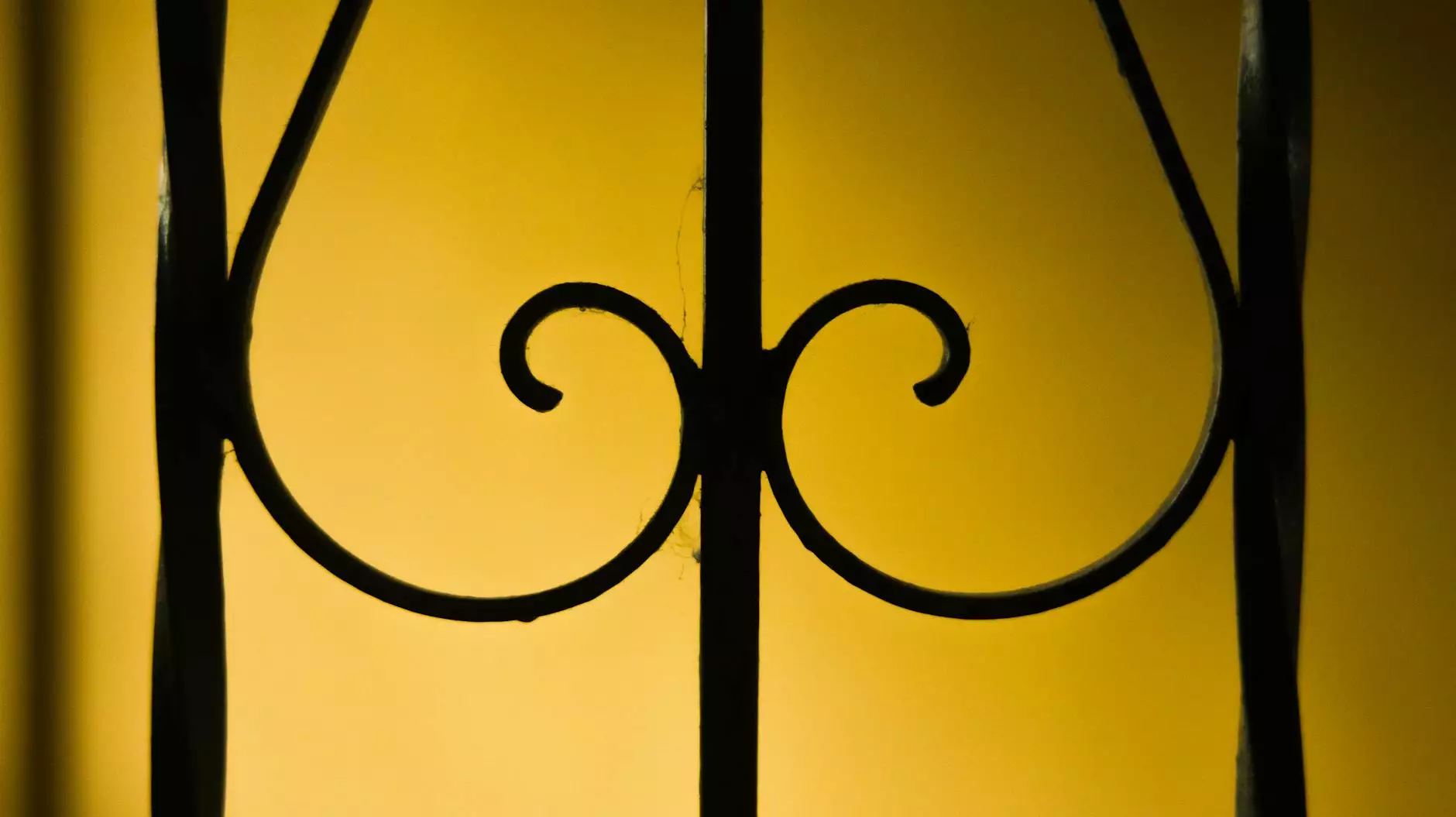
Autonomous driving represents the forefront of technological advancements in the automotive industry, offering unprecedented safety, efficiency, and convenience. At the heart of this innovation lies a powerful technique known as semantic segmentation. This article delves into the critical role of semantic segmentation in autonomous driving, providing insights into its applications, benefits, and the importance of data annotation tools in enhancing the accuracy of this process.
Understanding Semantic Segmentation in Autonomous Driving
Semantic segmentation is a process in computer vision that involves classifying each pixel in an image into a specific category. In the context of autonomous driving, this means identifying and labeling various components within the vehicle's visual field—such as cars, pedestrians, bicycles, road signs, and road lanes.
How Semantic Segmentation Works
The essence of semantic segmentation lies in its ability to create a detailed understanding of the environment. By analyzing images from cameras mounted on vehicles, semantic segmentation algorithms can process the information and generate a pixel-wise mask for each recognized object. Here's a quick overview of how it works:
- Data Collection: High-quality images and video data are collected from various sources, including cameras and LiDAR sensors.
- Data Annotation: Each object within these images is manually or automatically annotated, allowing algorithms to learn the characteristics of different segments.
- Model Training: Machine learning models are trained on this annotated data, enabling them to predict labels for unseen images.
- Real-Time Processing: During driving, the trained model processes incoming video feeds, providing real-time feedback on the environment.
The Importance of Data Annotation Tools
The accuracy of semantic segmentation models heavily depends on the quality and quantity of the annotated data. This is where specialized data annotation tools come into play. Platforms like KeyLabs.ai are designed to facilitate precise and efficient data annotation, ensuring that machine learning models are trained on high-quality datasets.
Benefits of Semantic Segmentation in Autonomous Driving
Implementing semantic segmentation in autonomous driving systems yields a multitude of benefits:
- Enhanced Object Recognition: Semantic segmentation improves the vehicle’s ability to recognize and differentiate between various objects on the road.
- Improved Decision-Making: By accurately identifying entities in the driving environment, autonomous systems can make better-informed decisions, such as when to stop or accelerate.
- Increased Safety: With the precise identification of pedestrians and obstacles, semantic segmentation contributes to safer driving experiences.
- Realistic Environment Modeling: Semantic segmentation aids in constructing detailed 3D maps of the environment, enhancing the vehicle’s spatial awareness.
Challenges in Semantic Segmentation for Autonomous Driving
Despite its advantages, several challenges persist in the adoption of semantic segmentation within autonomous driving applications:
- Data Quality: The performance of segmentation models heavily relies on high-quality annotated datasets. Variability in data quality can lead to inaccuracies.
- Computational Complexity: Real-time processing of high-resolution images requires significant computational power, which can introduce latency in decision-making.
- Environmental Variability: The models must be robust enough to handle various weather conditions, lighting scenarios, and complex urban environments.
The Role of KeyLabs.ai in Enhancing Semantic Segmentation
KeyLabs.ai stands at the forefront of providing superior data annotation platforms that specifically cater to the rigorous demands of semantic segmentation in autonomous driving. By utilizing advanced technology and a team of skilled annotators, KeyLabs.ai ensures the following:
Precision in Data Annotation
The platform uses state-of-the-art tools that enable precise labeling of images, thereby ensuring that every detail is captured. This level of precision is essential for training robust models capable of operating in real-world scenarios.
Scalability and Efficiency
KeyLabs.ai is designed to handle large volumes of data efficiently, which allows for rapid turnaround times without compromising quality. This is crucial in industries where timely data annotation can significantly impact project timelines.
Quality Control Mechanisms
With built-in quality assurance processes, KeyLabs.ai ensures that all annotated data meets stringent quality standards. This minimizes the potential for errors in model training and supports higher accuracy in predictions.
Future Trends in Semantic Segmentation for Autonomous Driving
The field of semantic segmentation is constantly evolving, and several trends are emerging that will shape its future in autonomous driving:
- Integration of AI and Machine Learning: Continued advancements in AI and ML techniques will enhance the accuracy and efficiency of semantic segmentation models.
- Use of Synthetic Data: Generating synthetic datasets for training can help mitigate the challenges of data scarcity and variability.
- Self-Supervised Learning: New approaches in self-supervised learning may reduce the dependence on labeled data, streamlining the annotation process.
- Cross-Domain Transfer: Techniques to improve model generalization across different environments will be critical for widespread operational effectiveness.
Conclusion
As we steer towards a future dominated by autonomous vehicles, the role of semantic segmentation will be pivotal in ensuring the safety and effectiveness of these systems. Through sophisticated data annotation platforms like KeyLabs.ai, businesses can harness the potential of semantic segmentation, contributing to advancements in autonomous driving technology. By embracing these modern tools and techniques, we can anticipate a safer, more efficient, and technologically advanced driving experience in the near future.
In summary, the scope of semantic segmentation extends far beyond mere object recognition; it is integral to the foundational systems that will drive the next generation of vehicles. As the industry progresses, the collaborative efforts between technology providers and data annotation experts will be crucial in overcoming challenges and fulfilling the promises of autonomous driving.
semantic segmentation autonomous driving